Minsu Chang
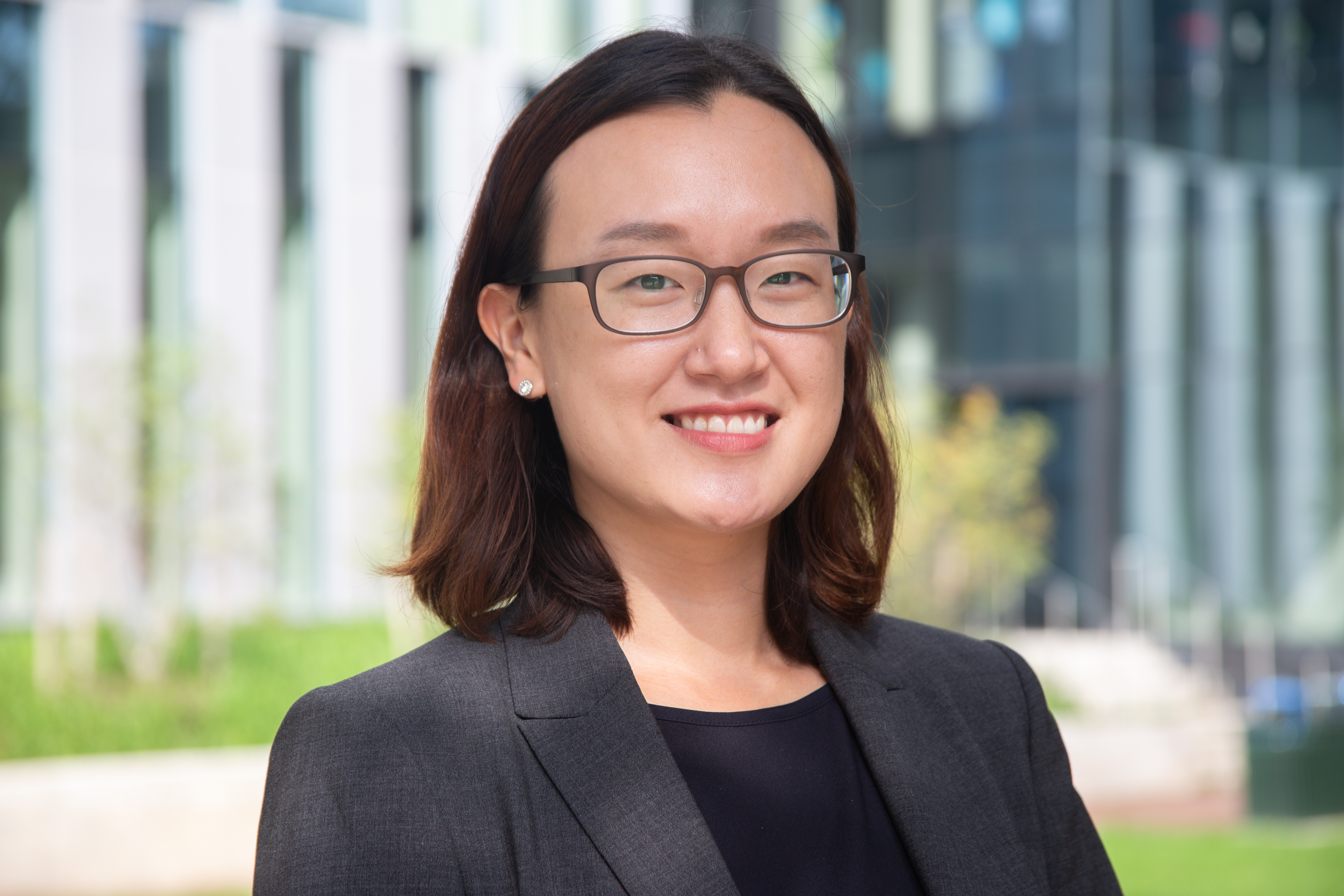
A House Without a Ring: The Role of Changing Marital Transitions for Housing Decisions
This paper shows that the evolving likelihood of marriage and divorce is an essential factor in accounting for the changes in housing decisions over time in the United States. To quantify the importance of this channel, I build a life-cycle model of single and married households who face exogenous age-dependent marital transition shocks. I then estimate the parameters of the model by a limited information Bayesian method to match the moments from 1995's cross-section data. I conduct a decomposition analysis between 1970 and 1995, two years with similar real house prices but substantially different probabilities of marital transitions. I find that the change in the likelihood of marital transitions accounts for 29% of the observed increase in the homeownership rate of singles. This portion is substantial given that the changes in downpayment requirements, earnings risk, and spousal labor productivity jointly replicate 45% of the change. When the change in marital transitions is shut down, the marrieds' housing asset share increases, which is opposite to the data's pattern. Then I extend my analysis to study whether the ongoing change in marital transitions still plays a role in explaining housing decisions in recent years, which have seen dramatically changing house prices. In addition to other factors such as credit constraints, wages, and beliefs on price appreciation that are often suggested as drivers for homeownership increase during the housing boom in the mid-2000s, I show that the continuing decrease in marriage contributes to an approximately 7% increase in the homeownership rate for young singles.
Heterogeneity and Aggregate Fluctuations (with Xiaohong Chen and Frank Schorfheide)
We develop a state-space model with a state-transition equation that takes the form of a functional vector autoregression and stacks macroeconomic aggregates and a cross-sectional density. The measurement equation captures the error in estimating log densities from repeated cross-sectional samples. The log densities and the transition kernels in the law of motion of the states are approximated by sieves, which leads to a finite-dimensional representation in terms of macroeconomic aggregates and sieve coefficients. We use this model to study the joint dynamics of technology shocks, per capita GDP, employment rates, and the earnings distribution. We find that the estimated spillovers between aggregate and distributional dynamics are generally small, a positive technology shocks tends to increase the fraction of individuals earning less than the labor share of per capita GDP, and shocks that raise the inequality of earnings have ambiguous effects on per-capita GDP.
Bypassing the Curse of Dimensionality: Feasible Multivariate Density Estimation (with Paul Sangrey)
Most economic data are multivariate and so estimating multivariate densities is a classic problem in the literature. However, given vector-valued data, the curse of dimensionality makes nonparametrically estimating the data’s density infeasible if the number of series D is large. Hence, we do not seek to provide estimators that perform well all of the time (it is impossible), but rather seek to provide estimators that perform well most of the time. We adapt the ideas in the Bayesian compression literature to this environment by randomly binning the data. The binning randomly determines both the number of bins and which observation is placed in which bin. This novel procedure induces a simple mixture representation for the data’s density. For any finite number of periods T, the number of mixture components used is random. We construct a bound for this variable as a function of T that holds with high probability. We adopt the nonparametric Bayesian framework and construct a computationally efficient density estimator using Dirichlet processes. Since the number of mixture components is the key determinant of our model’s complexity, our estimator’s convergence rates — √log(T)/√T in the unconditional case and log(T)/√T in the conditional case — depend on D only through the constant term. We then analyze our estimators' performance in a monthly macroeconomic panel and a daily financial panel. Our procedure performs well in capturing the data’s stylized features such as time-varying volatility and fat-tails.
PIER Workshop on Quantitative Tools for Macroeconomics, University of Pennsylvania, Lab Instructor (Spring 2018, Spring 2017)
Statistics for Economists (Undergraduate), University of Pennsylvania, Instructor (Summer 2017)
Econometrics I (Graduate), University of Pennsylvania, Teaching Assistant for Professor Frank Schorfheide and Professor Xu Cheng (Fall 2015, Fall 2014)
Econometrics (Undergraduate), University of Pennsylvania, Teaching Assistant for Professor Frank Diebold (Spring 2015)
Publications
“A Generalized Focused Information Criterion for GMM with Applications to Panel Data Models,” with Francis J. DiTraglia, Journal of Applied Econometrics, 2018, 33 (3), pp. 378-397.
“Nonparametric Tests of Conditional Treatment Effects with an Application to Single-Sex Schooling on Academic Achievements,” with Sokbae (Simon) Lee and Yoon-Jae Whang, The Econometrics Journal, 2015, 18 (3), pp. 307-346.
Quantitative Macroeconomics, Econometrics
Jesus Fernandez-Villaverde
Frank Schorfheide
Professor Jesus Fernandez-Villaverde (Co-advisor)
133 South 36th Street, Office 521
Philadelphia, PA 19104
215-898-1504
Professor Frank Schorfheide (Co-advisor)
133 South 36th Street, Office 621
Philadelphia, PA 19104
215-898-8486
Professor Francis J. DiTraglia
133 South 36th Street, Office 630
Philadelphia, PA 19104
215-898-1506
Professor Dirk Krueger
133 South 36th Street, Office 520
Philadelphia, PA 19104
215-573-1424